Misinformation
The misinformation project characterizes and identifies misinformation and fake news on social media in order to develop approaches for mitigating its spread.
Given the increase in fake news, it is crucial to examine the content of fake news content relevant to U.S. politics and COVID-19, understand its appeal, and build models of diffusion to predict and eventually limit its spread.
Low-Quality Misinformation COVID-19 Sources Network Clustering Using Modularity.
Political Misinformation
During the 2016 election, misinformation spread about both candidates throughout the campaign. The figure to the right shows the proportion of fake news on Twitter about both candidates from June, 2016 through November, 2016. Expanding on the work done in 2016, the project team continued to track misinformation for the 2020 election.
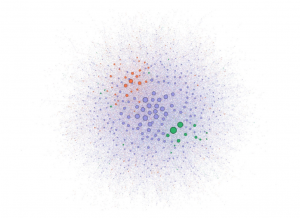
Network of Traditional News Sources (purple), High-Quality Health Sources (green), and Low-Quality/Misinformation Sources (Orange)
COVID-19 Misinformation
The project team is investigating what COVID-19 misinformation is being shared online by examining the links being shared on Twitter on posts about COVID-19. While Twitter users share links to high-quality information sources slightly less than misinformation and fake news sources, there is a well connected network of low-quality COVID-19 related information on the web, and both high-quality health and news sources are connecting to this community.
Project Team
The research team conducting analysis of the results of this project are Leticia Bode and Lisa Singh from Georgetown University and Ceren Budak from the University of Michigan. Georgetown student Kornraphop (Ken) Kawintiranon also assists with this research.
Publications
Kawintiranon, K., Singh, L., & Budak, C. (2022). Traditional and context-specific spam detection in low resource settings. Machine Learning, https://link.springer.com/article/10.1007/s10994-022-06176-x.
Kawintiranon, K. & Singh, L. (2022). DeMis: Data-efficient misinformation detection using reinforcement learning. In Proceedings of the European Conference on Machine Learning and Principles and Practice of Knowledge Discovery in Databases. Grenoble, France.
Singh, L., Bansal, S., Bode, L., Budak, C., Chi, G., Kawintiranon, K., … & Wang, Y. (2020). A first look at COVID-19 information and misinformation sharing on Twitter. arXiv preprint arXiv:2003.13907.
Budak, C. (2019, May). What happened? the spread of fake news publisher content during the 2016 us presidential election. In The World Wide Web Conference (pp. 139-150).
Recent Work
Understanding high- and low-quality URL Sharing on COVID-19 Twitter streams
This article investigates the prevalence of high and low quality URLs shared on Twitter when users discuss COVID-19. Read more in the Journal of Computational Social Science.
Misinformation and the Impact of Social Media in Elections
Lisa Singh and Ceren Budak presented virtually for an event hosted by the McCourt school's Massive Data Institute and the Institute of Politics and Public Service (GU Politics) about the impact of misinformation in the 2016 election and what it could mean for the...
Twitter and COVID-19: Preliminary Study Finds Information and Misinformation Equally Shared
Read more on Georgetown's McCourt School of Public Policy website.